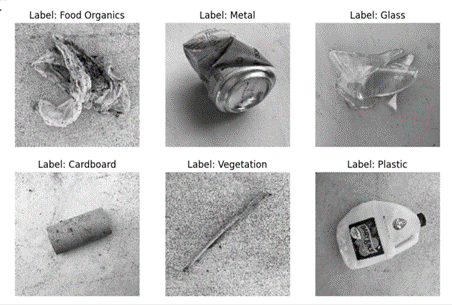
The increasingly complex waste problem in Indonesia can be attributed to the lack of public awareness about the importance of waste management and changes in production and consumption patterns. To address this issue, UGM has developed a research roadmap focusing on Climate Change and Environmental Adaptation, aligning with the Sustainable Development Goals (SDGs) related to Responsible Consumption and Production. A digital transformation utilizing mobile computing technology and smart devices, particularly the Internet of Things (IoT), is essential for a fast, accurate, and effective solution.
In this research, Dr. Yuris Mulya Saputra, a lecturer from the Department of Electrical Engineering and Informatics at SV UGM, through the SV UGM Community Research Fund 2024, proposes the use of transfer learning (TL) on IoT devices as an efficient solution for waste management, particularly in detecting and sorting waste types. TL is a machine learning approach that allows each user device to independently train on waste type images without the need for data sharing. A model trained with high accuracy on a cloud server will be transferred to user devices, enabling independent training even with limited image data.
The primary focus of this research is to improve waste detection accuracy using TL on IoT devices with limited computing resources. To achieve accurate waste classification, a deep learning method based on convolutional neural networks will be employed. This research is expected to produce a TL-based image classification system that is simple yet effective, allowing IoT device users to sort waste while maintaining their data privacy.